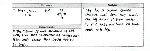
Unveiling Students' Understanding of Ammonia as a Weak Base through Scaffolding-Based Chemical Reasoning Assessment
Abstract
Reasoning is a basic cognitive ability in science learning, especially in chemistry, in which students must connect macroscopic, symbolic, and microscopic levels. However, most students seem to have difficulty learning chemical reasoning, especially in the ionization of weak bases (examples: NH₃). This study uses a scaffold-based assessment to evaluate students' explanations for ammonia as a base. A paper-and-pencil test was applied to 91 first-year preservice chemistry students to test them on phenomenological, mechanical, and structural types of reasoning. Two raters rated responses, and scoring reliability was assessed using Cohen’s Kappa (0.925). The data analysis consisted of descriptive statistics, correlation analysis, clustering (K-Means and t-SNE), and regression prediction with XGBoost. The results demonstrate that structural reasoning exhibits the highest level, but phenomenological reasoning has the most variation. There appears to be a high correlation between phenomenological empirical generalization and structural reasoning (r = 0.35+). Clustering outputs show three categories of students: high (R3), moderate (R2), and low (R1) reasoning, and most of the students are categorized at the moderate reasoning level, indicating some misconceptions. The XGBoost model performs well in predicting high-reasoning students but not in the moderate-reasoning classification. This paper indicates the power of scaffolding-embedded assessment for deducing reasoning patterns and misconceptions in ammonia ionization. The results can guide adaptive learning approaches for improving students' chemical reasoning.
Keywords
Full Text:
PDFReferences
[1] M. Weinrich and V. Talanquer, “Mapping Students’ Modes of Reasoning When Thinking About Chemical Reactions Used to Make a Desired Product,” Chem. Educ. Res. Pract., vol. 17, no. 2, pp. 394–406, 2016, doi:10.1039/c5rp00208g.
[2] T. Nkosi and L. Mnguni, “The Impact of Physical Molecular Models on Students’ Visuo-Semiotic Reasoning Skills Related to the Lewis Structure and Ball-and-Stick Model of Ammonia,” J. Balt. Sci. Educ., vol. 19, no. 4, pp. 594–604, 2020, doi:10.33225/jbse/20.19.594.
[3] M. M. Cooper, H. Kouyoumdjian, and S. M. Underwood, “Investigating Students’ Reasoning About Acid–Base Reactions,” J. Chem. Educ., vol. 93, no. 10, pp. 1703–1712, 2016, doi:10.1021/acs.jchemed.6b00417.
[4] G. Bhattacharyya, “From Source to Sink: Mechanistic Reasoning Using the Electron-Pushing Formalism,” J. Chem. Educ., vol. 90, no. 10, pp. 1282–1289, 2013, doi:10.1021/ed300765k.
[5] V. Talanquer, “The Complexity of Reasoning About and With Chemical Representations,” JACS Au, vol. 2, no. 12, pp. 2658–2669, 2022, doi:10.1021/jacsau.2c00498.
[6] N. J. Kim, B. R. Belland, and A. Walker, “Effectiveness of Computer-Based Scaffolding in the Context of Problem-Based Learning for STEM Education: Bayesian Meta-Analysis,” Educ. Psychol. Rev., vol. 30, no. 2, pp. 397–429, 2017, doi:10.1007/s10648-017-9419-1.
[7] B. R. Belland, A. Walker, N. J. Kim, and M. Lefler, “Synthesizing Results From Empirical Research on Computer-Based Scaffolding in STEM Education,” Rev. Educ. Res., vol. 87, no. 2, pp. 309–344, 2016, doi:10.3102/0034654316670999.
[8] Z. D. Kırbulut and Ö. Geban, “Using Three-Tier Diagnostic Test to Assess Students’ Misconceptions of States of Matter,” Eurasia J. Math. Sci. Technol. Educ., vol. 10, no. 5, 2014, doi:10.12973/eurasia.2014.1128a.
[9] P. Zhang and F. Zhang, “Enhancing Education and Teaching Management Through Data Mining and Support Vector Machine Algorithm,” Int. J. e-Collaboration, vol. 20, pp. 1–16, Oct. 2024, doi:10.4018/IJeC.357998.
[10] R. A. Triwardani, Y. Fuad, and M. Masriyah, “Students’ Reasoning Behavior on Generalization of Figural Pattern,” 2018, doi:10.2991/miseic-18.2018.62.
[11] E. Lintunen et al., “Cognitive abilities predict performance in everyday computer tasks,” Int. J. Hum. Comput. Stud., vol. 192, p. 103354, 2024, doi:10.1016/j.ijhcs.2024.103354.
[12] N. Zaini and N. Rahmat, “Exploring the Relationship between Learning Strategies Used in Language Learning,” Int. J. Acad. Res. Bus. Soc. Sci., vol. 13, pp. 2556–2572, May 2023, doi:10.6007/IJARBSS/v13-i5/17030.
[13] Y. Yuberti, Y. Suryani, and I. Kurniawati, “Four-Tier Diagnostic Test With Certainty of Response Index to Identify Misconception in Physics,” Indones. J. Sci. Math. Educ., vol. 3, no. 2, pp. 245–253, 2020, doi:10.24042/ijsme.v3i2.6061.
[14] N. Malik, N. Sangwan, N. Bohra, A. Kumari, D. Sheoran, and M. Dabas, “HyperSegRec: enhanced hypergraph-based recommendation system with user segmentation and item similarity learning,” Cluster Comput., vol. 27, pp. 11727–11745, Jun. 2024, doi:10.1007/s10586-024-04560-x.
[15] T. Gong, Y. Jiang, L. Saldivia, and C. Agard, “Using Sankey diagrams to visualize drag and drop action sequences in technology-enhanced items,” Behav. Res. Methods, vol. 54, Jun. 2021, doi:10.3758/s13428-021-01615-4.
[16] A. Asselman, M. Khaldi, and A. Souhaib, “Enhancing the prediction of student performance based on the machine learning XGBoost algorithm,” Interact. Learn. Environ., vol. 31, pp. 1–20, May 2021, doi:10.1080/10494820.2021.1928235.
[17] F. V. Ryzhkov, Y. E. Ryzhkova, and M. N. Elinson, “Python in Chemistry: Physicochemical Tools,” Processes, vol. 11, no. 10, p. 2897, 2023, doi:10.3390/pr11102897.
[18] J. Lönnbro, L. D. Holmqvist, E. Persson, P. Thysell, N. D. Åberg, and S. M. Wallerstedt, “Inter‐rater Reliability of Assessments Regarding the Quality of Drug Treatment, and Drug‐related Hospital Admissions,” Br. J. Clin. Pharmacol., vol. 87, no. 10, pp. 3825–3834, 2021, doi:10.1111/bcp.14790.
[19] J. R. Landis and G. G. Koch, “The Measurement of Observer Agreement for Categorical Data,” Biometrics, vol. 33, no. 1, pp. 159–174, Apr. 1977, doi:10.2307/2529310.
[20] I. N. Suastika and A. A. I. D. A. Utami, “Test the Validity of the Multicultural Education Course at the E-Learning (Moodel Learning Management System) Universitas Pendidikan Ganesha,” 2023, doi:10.4108/eai.28-10-2022.2326354.
[21] T. C. Postma and J. G. White, “Developing Integrated Clinical Reasoning Competencies In Dental Students Using Scaffolded Case‐Based Learning – Empirical Evidence,” Eur. J. Dent. Educ., vol. 20, no. 3, pp. 180–188, 2015, doi:10.1111/eje.12159.
[22] M. M. Cooper, L. M. Corley, and S. M. Underwood, “An Investigation of College Chemistry Students’ Understanding of Structure–Property Relationships,” J. Res. Sci. Teach., vol. 50, no. 6, pp. 699–721, 2013, doi:10.1002/tea.21093.
[23] A. Kondinski, J. Bai, S. Mosbach, J. Akroyd, and M. Kraft, “Knowledge Engineering in Chemistry: From Expert Systems to Agents of Creation,” Acc. Chem. Res., vol. 56, no. 2, pp. 128–139, 2022, doi:10.1021/acs.accounts.2c00617.
[24] J. Pelamonia and A. D. Corebima, “Cognitive Basis and Semantic Structure of Phenomenological Reasoning on Science Among Lower Secondary School Students: A Case of Indonesia,” J. Balt. Sci. Educ., vol. 14, no. 4, pp. 474–486, 2015, doi:10.33225/jbse/15.14.474.
[25] I. Medhi, M. Lakshmanan, K. Toyama, and E. Cutrell, “Some Evidence for the Impact of Limited Education on Hierarchical User Interface Navigation,” 2013, doi:10.1145/2470654.2481390.
[26] J. Olander, C. Stenberg, S. Stenlund, and M. Andrée, “Didactic Reasoning About Using Chemicals in Teaching Upper Secondary Chemistry,” J. Chem. Educ., vol. 100, no. 1, pp. 45–52, 2022, doi:10.1021/acs.jchemed.2c00511.
[27] K. Heisterkamp and V. Talanquer, “Interpreting Data: The Hybrid Mind,” J. Chem. Educ., vol. 92, no. 12, pp. 1988–1995, 2015, doi:10.1021/acs.jchemed.5b00589.
[28] K. S. Taber, “Revisiting the Chemistry Triplet: Drawing Upon the Nature of Chemical Knowledge and the Psychology of Learning to Inform Chemistry Education,” Chem. Educ. Res. Pract., vol. 14, no. 2, pp. 156–168, 2013, doi:10.1039/c3rp00012e.
[29] G. Chittleborough and D. F. Treagust, “The Modelling Ability of Non-Major Chemistry Students and Their Understanding of the Sub-Microscopic Level,” Chem. Educ. Res. Pract., vol. 8, no. 3, pp. 274–292, 2007, doi:10.1039/b6rp90035f.
[30] A. J. Dood and F. M. Watts, “Students’ Strategies, Struggles, and Successes With Mechanism Problem Solving in Organic Chemistry: A Scoping Review of the Research Literature,” J. Chem. Educ., vol. 100, no. 1, pp. 53–68, 2022, doi:10.1021/acs.jchemed.2c00572.
[31] M. B. Permatasari, S. Rahayu, and I. W. Dasna, “Chemistry Learning Using Multiple Representations: A Systematic Literature Review,” J. Sci. Learn., vol. 5, no. 2, pp. 334–341, 2022, doi:10.17509/jsl.v5i2.42656.
[32] T. R. Besold, A. S. d. Garcez, K. Stenning, L. v. d. Torre, and M. v. Lambalgen, “Reasoning in Non-Probabilistic Uncertainty: Logic Programming and Neural-Symbolic Computing as Examples,” Minds Mach., vol. 27, no. 1, pp. 37–77, 2017, doi:10.1007/s11023-017-9428-3.
[33] K. Kamaluddin, N. K. Sani, I. W. Darmadi, and N. Nurgan, “Analysis of Students Scientific Reasoning Ability and the Correlation to Students Cognitive Ability in Physics Learning,” J. Penelit. Pendidik. Ipa, vol. 9, no. 11, pp. 10173–10179, 2023, doi:10.29303/jppipa.v9i11.5657.
[34] D. B. Haas, F. M. Watts, A. J. Dood, and G. V. Shultz, “Analysis of Organic Chemistry Students’ Developing Reasoning Elicited by a Scaffolded Case Comparison Activity,” Chem. Educ. Res. Pract., vol. 25, no. 3, pp. 742–759, 2024, doi:10.1039/d4rp00021h.
[35] L. Keiner and N. Graulich, “Transitions Between Representational Levels: Characterization of Organic Chemistry Students’ Mechanistic Features When Reasoning About Laboratory Work-Up Procedures,” Chem. Educ. Res. Pract., vol. 21, no. 1, pp. 469–482, 2020, doi:10.1039/c9rp00241c.
[36] N. Becker, C. Stanford, M. H. Towns, and R. S. Cole, “Translating Across Macroscopic, Submicroscopic, and Symbolic Levels: The Role of Instructor Facilitation in an Inquiry-Oriented Physical Chemistry Class,” Chem. Educ. Res. Pract., vol. 16, no. 4, pp. 769–785, 2015, doi:10.1039/c5rp00064e.
[37] M. A. Syakur, B. K. Khotimah, E. M. S. Rochman, and B. D. Satoto, “Integration K-Means Clustering Method and Elbow Method for Identification of the Best Customer Profile Cluster,” IOP Conference Series: Materials Science and Engineering, vol. 336, p. 012017, 2018, doi:10.1088/1757-899X/336/1/012017.
[38] C. Shi, B. Wei, S. Wei, W. Wang, H. Liu, and J. Liu, “A Quantitative Discriminant Method of Elbow Point for the Optimal Number of Clusters in Clustering Algorithm,” EURASIP Journal on Wireless Communications and Networking, vol. 2021, no. 1, 2021, doi:10.1186/s13638-021-01910-w.
[39] M. Charrad, N. Ghazzali, V. Boiteau, and A. Niknafs, “NbClust: An R Package for Determining the Relevant Number of Clusters in a Data Set,” Journal of Statistical Software, vol. 61, no. 6, 2014, doi:10.18637/jss.v061.i06.
[40] C.-I. Lee, Y.-F. Yang, and S.-Y. Mai, “The Impact of a Scaffolded Assessment Intervention on Students’ Academic Achievement in Web-Based Peer Assessment Activities,” International Journal of Distance Education Technologies, vol. 14, no. 4, pp. 41–54, 2016, doi:10.4018/ijdet.2016100104.
[41] L. Hamerská, T. Matěcha, M. Tóthová, and M. Rusek, “Between Symbols and Particles: Investigating the Complexity of Learning Chemical Equations,” Education Sciences, vol. 14, no. 6, p. 570, 2024, doi:10.3390/educsci14060570.
[42] A. Wiyarsi, A. R. Fachriyah, D. Supriadi, and M. I. B. M. Damanhuri, “A Test of Analytical Thinking and Chemical Representation Ability on ‘Rate of Reaction’ Topic,” Jurnal Cakrawala Pendidikan, vol. 38, no. 2, pp. 228–242, 2019, doi:10.21831/cp.v38i2.23062.
[43] P. D. Natalia and A. Sudrajat, “Development of Three-Tier Diagnostic Test Instrument to Measure Misconceptions of Class XI Students on Reaction Rate Materials,” Jurnal Teknologi Pendidikan, vol. 8, no. 1, p. 1, 2023, doi:10.33394/jtp.v8i1.6192.
[44] F. Mardika and A. Mahmudi, “An Analysis of Proportional Reasoning Ability of Junior High School Students,” Jurnal Riset Pendidikan Matematika, vol. 8, no. 1, pp. 22–32, 2021, doi:10.21831/jrpm.v8i1.14995.
[45] G. D. Y. Kumlu and N. Yürük, “Investigation of Cognitive and Metacognitive Strategies Used by Preservice Science Teachers Exposed to Explicit and Peer Tutoring Reading Strategies Instruction,” International Journal of Contemporary Educational Research, vol. 10, no. 1, pp. 1–24, 2023, doi:10.33200/ijcer.1082537.
[46] H. Herunata, I. M. Octavia, H. W. Wijaya, and P. Parlan, “Correlational Analysis of Conceptual Understanding, Chemical Representation, and Representational Competence on Acid-Base,” 2023, doi:10.1063/5.0112177.
[47] S. B. S. Lai, N. H. N. B. M. Shahri, M. Mohamad, H. A. B. A. Rahman, and A. Rambli, “Comparing the Performance of AdaBoost, XGBoost, and Logistic Regression for Imbalanced Data,” Mathematics and Statistics, vol. 9, no. 3, pp. 379–385, 2021, doi:10.13189/ms.2021.090320.
[48] P. Zhang, Y. Jia, and Y. Shang, “Research and Application of XGBoost in Imbalanced Data,” International Journal of Distributed Sensor Networks, vol. 18, no. 6, p. 155013292211069, 2022, doi:10.1177/15501329221106935.
[49] A. Jufriadi, H. D. Ayu, and H. Y. Pratiw, “Developing E-Scaffolding Integrated With E-Assessment to Improve Students’ Mastery of Concept,” 2019, doi:10.2991/icesre-18.2019.37.
[50] M. Dobber, R. Zwart, M. Tanis, and B. van Oers, “Literature Review: The Role of the Teacher in Inquiry-Based Education,” Educational Research Review, vol. 22, pp. 194–214, 2017, doi:10.1016/j.edurev.2017.09.002.
Refbacks
- There are currently no refbacks.